Volume 8 Issue 1 (2010)
DOI:10.1349/PS1.1537-0852.A.377
Note: Linguistic Discovery uses Unicode characters
to represent phonetic symbols. Please see Optimizing Display
for requirements to accurately reproduce this page.
Drawing Networks from Recurrent Polysemies
Comment on ‘Polysemous Qualities and Universal
Networks’ by Loïc-Michel Perrin (2010)
Michael Cysouw
Max Planck Institute for Evolutionary Anthropology,
Leipzig
Recurrent polysemy across languages is a very powerful
source of information for studying semantic similarity. The data as collected by
Perrin (2010) provide a fascinating basis for inquiries into the relations
between meanings of adjective-like predicates, or ‘qualities’, as
Perrin calls them. Unfortunately, I think that the real potential of his data
does not become apparent from the way the data are analyzed and presented in his
paper. In this comment, I will offer a different possibility for graphical
display of the data from Perrin’s paper. This comment is in no way
intended to be the definitive answer to the problem of visualizing
cross-linguistic data. However, I hope that it will inspire linguists to look
more closely into the extensive literature on data visualization to find
suitable methods for any concrete problem at hand.
Before I will turn to the question of visualization, I would like to
briefly address two other aspects of Perrin’s paper that I consider
debatable. First, there is the problem of cross-linguistic invariants. The
concepts investigated by Perrin are defined by their English, French and German
translations. Although taking three languages is of course more precise than
just taking one as the defining meta-language, such a definition of
cross-linguistic concepts still allows for a large space of variation. For
example, as Perrin himself notes in section 2.2, one could find more than 30
translations of the English word
dry in French. Taking the
English/French/German triad of words
dry/sec/trocken as a definition of a
cross-linguistic concept still underdetermines the precise meaning intended. For
future projects of this kind, I would strongly urge to use a
contextually-embedded definition of the cross-linguistic concepts, e.g.
exemplified by a sentence or a small paragraph in which the intended meaning
occurs. So, instead of looking for the somewhat ominous translation of
dry/sec/trocken,
one would look for the translation of a word in a
context, like in French
“fruit
sec”
or in English
“hot and
dry weather is expected to persist today through
Friday
” (cf. Cysouw 2010; Wälchli 2010).
Second, there is the problem of statistical assessment of the attested
frequencies. It is always difficult to deal with the large variation in
cross-linguistic studies, but I consider arbitrary divisions of a scale not a
very suitable means for interpretative practice. Why, for example, are
federative notions defined as “qualities which are involved in a minimum
of five polysemous patterns and across a minimum of six languages” (Perrin
2010: section 4.2)? Why five patterns and six languages? Why not two, or three,
or ten? Or why not across at least three different genera or families? There is
no easy answer to these questions, but I would urge all analysts of
cross-linguistic data to try and avoid such all-or-nothing categorizations and
think more along lines of continuous clines of gradual variation.
Finally then, let me turn to the visualization of recurrent polysemies.
In the received approach to semantic maps, as presented concisely in Haspelmath
(2003), all attested polysemy is taken into account for drawing a network. As
criticized in Cysouw (2007), in that approach too much importance is given to
incidentally-occurring polysemies relative to the much more interesting
frequently-occurring ones. Perrin uses a minimum of three languages for a
polysemy to be drawn on the map (section 4.1). Again, the question arises: why
three languages? Why not two, or ten? Further, in the methodology for semantic
maps as described by Haspelmath (2003), there is no mechanism proposed for how
to organize the network of a semantic map, other than that the graph should be
preferably planar (i.e. there should be no crossing lines). As a solution to the
problem of visualizing such data, it has been proposed in various recent papers
on semantic maps to use methods like multi-dimensional scaling (MDS) instead of
a graph-based approach (Cysouw 2001; Levinson & Meira 2003; Cysouw 2007;
Croft & Poole 2008; Wälchli 2010). However, it turns out that the
semantic structure of lexical domains is often highly multidimensional, which
renders MDS suboptimal for graphical display (Wälchli and Cysouw 2008). For
that reason, I will propose here to visualize Perrin’s data by using one
of the many other methods for graphical display as developed in the past
decades. The networks shown below were produced by the Fruchterman-Reingold
graph-layout algorithm (Fruchterman and Reingold 1991). In this algorithm, the
lines between two concepts are considered to be springs, which pull concepts
closer together the more often a particular polysemy is attested in the
data.[1]
A suitable layout is then
computed which attempts to minimize the strain on the connections between
concepts.
Figure 1 shows the network when including all polysemies as listed in
Appendix 2 of Perrin’s paper. The length of the lines is approximately
inversely proportional to the frequency of attestation (i.e. the longer a line,
the less common the polysemy). However, as can be seen in the figure, there is a
bewildering extent of variation of polysemies in the data, leading to a not very
useful display with many crossing lines. Note, though, that the display still
includes some information in the form of clusters of closely positioned
concepts, like
small/little/young or
strong/solid/hard/gesund. The
concepts in these clusters correlate strongly with Perrin’s federative
notions.
Figure 1. Complete graph of all
polysemies
To reduce the amount of information in the graph (so that a
human can judge the content more easily), I removed all polysemies that occur
only once in Perrin’s data. As a result, many concepts were not linked to
any other concepts any more, and I have removed all these from the display. The
graph resulting from the remaining concepts comprises eight separate subgraphs,
as shown in Figure 2. There are five small graphs, connecting just two or three
concepts (displayed at the bottom right of the figure). More interesting are the
three larger graphs in the figure. First, the graph to the upper right shows the
recurrent polysemies in the realm of tastes and smells with negative
connotations, connected through the concepts
bad and
nasty at the
center. Second, the large graph on the left is a more complex combination of
small-dimension qualities at the bottom (centered around
small/narrow/weak) and a collection of mostly positively connoted
qualities at the top (centered around
good and
clean). The link
between these two parts is established through the concept
mou (English
soft, German
weich). The central chain of polysemies seems to form
a semantic continuum:
small – narrow – thin – weak – soft – sweet – good – clean.
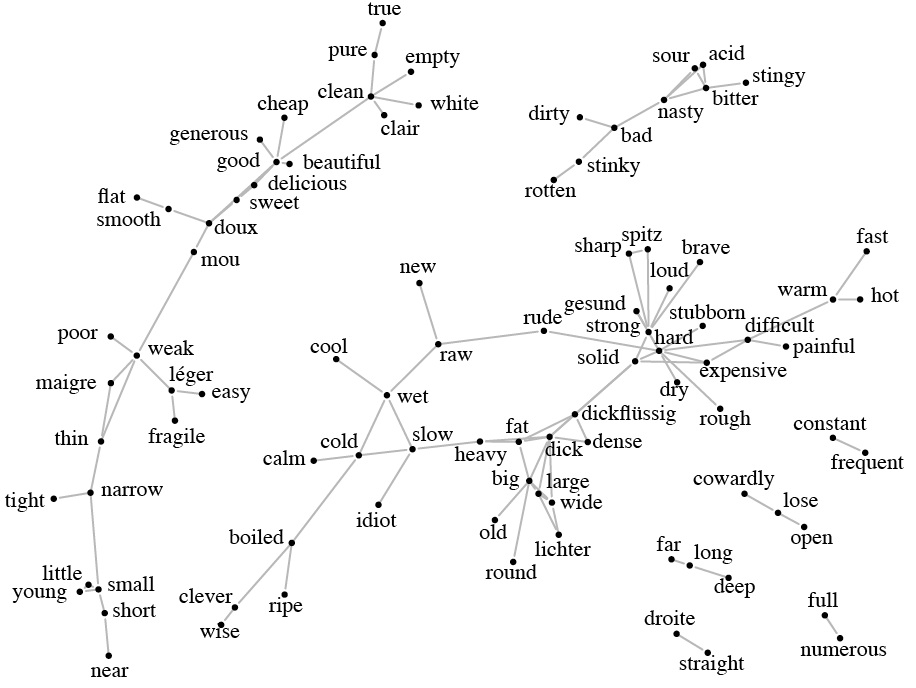
Figure 2. Graph of polysemies that occur in more than one
language
Finally, the largest graph is shown in the middle of Figure
2. This graph is still too complex to be displayed with reasonably true
distances and without crossing lines, so I removed the link between
difficult and
heavy to obtain at least an approximately planar
graph. The resulting graph shows two large clusters of concepts, one around
strong/hard/solid and another around
fat/big/thick. These two
clusters are directly linked by the polysemies
solid–dick,
solid–dickflüssig and
heavy–difficult (this last
one is not displayed in Figure 2). To the left side of this graph, there is a
somewhat less prominent chain of polysemies connecting these two main clusters
by the semantic continuum
hard – rude – raw – wet – cold – slow –
heavy
.
References
Croft, William and Keith T. Poole. 2008. Inferring universals from
grammatical variation: Multidimensional scaling for typological analysis.
Theoretical Linguistics 34/1.1-37. doi:10.1515/thli.2008.001
Cysouw, Michael. 2001. Review of Martin Haspelmath (1997)
‘Indefinite Pronouns’. Journal of Linguistics
37.99-114. doi:10.1017/s0022226701231351
-----. 2007. Building semantic maps: The case of person
marking. New challenges in typology, ed. by Bernhard Wälchli and Matti
Miestamo, 225-248. Berlin: Mouton de Gruyter. (Trends in Linguistics: Studies
and Monographs 189).
-----. 2010. Semantic maps as metrics on meaning.
Linguistic Discovery, this issue. doi:10.1349/ps1.1537-0852.a.346
Fruchterman, Thomas M. J. and Edward M. Reingold. 1991. Graph
drawing by force-directed placement. Software: Practice and Experience
21/11.1129-1164.
Gabor, Csardi and Nepusz Tamas. 2006. The igraph software package
for complex network research. InterJournal: Complex Systems 1695.
Haspelmath, Martin. 2003. The geometry of grammatical meaning:
Semantic maps and cross-linguistic comparison. The new psychology of language:
Cognitive and functional approaches to language structure, ed. by Michael
Tomasello, vol. 2, 211-242. Mahwah, NJ: Erlbaum.
Levinson, Stephen C. and Sérgio Meira. 2003. ‘Natural
concepts’ in the spatial topological domain - Adpositional meanings in
crosslinguistic perspective: An exercise in semantic typology. Language
79/3.485-516. doi:10.1353/lan.2003.0174
Perrin, Loïc-Michel. 2010. Polysemous qualities and universal
networks. Linguistic Discovery, this volume. doi:10.1349/ps1.1537-0852.a.353
R Development Core Team. 2008. R: A language and environment for
statistical computing. Vienna, Austria: R Foundation for Statistical
Computing.
Wälchli, Bernhard. 2010. Similarity semantics and building
probabilistic semantic maps from parallel texts. Linguistic Discovery, this
issue. doi:10.1349/ps1.1537-0852.a.356
Wälchli, Bernhard and Michael Cysouw. 2010. Lexical typology through similarity semantics: Toward a semantic map of motion verbs. Linguistics (forthcoming). doi:10.1515/ling-2012-0021
Author's contact information:
Michael Cysouw
Department of Linguistics
Max Planck Institute for Evolutionary Anthropology
Deutscher Platz 6
04103 Leipzig
Germany
cysouw@eva.mpg.de
[1]The graphs were drawn
by using the layout function
layout.fruchterman.reingold in the package
igraph (Gabor and Tamas 2006)
for the statistical program
R
(R Development Core Team 2008). It is not obvious how the frequency of
attestation should be translated into the ‘strength’ of the pull
between the concepts. For the illustrations shown here, I used the squared
frequencies as strength of the pull. Although this results in easily
interpretable pictures, I have no particular reason for this decision other than
the fact that it gives good results.
|